CAGIS Seminar Presents Tianyang Chen “Explicit Incorporation of Spatial Autocorrelation in 3D Deep Learning for Geospatial Object Detection”
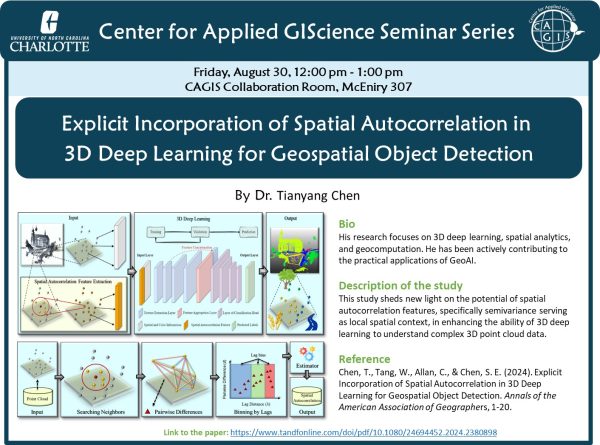
Please join CAGIS Seminar August, 30 at 12:00pm! For this seminar we will be hearing Dr. Tianyang Chen discuss his study: Explicit Incorporation of Spatial Autocorrelation in
3D Deep Learning for Geospatial Object Detection
Dr. Chen’s research focuses on 3D deep learning, spatial analytics, and geocomputation. He has been actively contributing to the practical applications of GeoAI.
Abstract
Three-dimensional (3D) geospatial object detection has become essential for 3D geospatial studies driven by explosive growth in 3D data. It is extremely labor- and cost-intensive, though, as it often requires manual detection. Deep learning has been recently used to automate object detection within 3D context. Yet, addressing spatial dependency in 3D data and how it might inform deep learning for 3D geospatial object detection remains a significant challenge. Traditional models focus on the use of spatial properties, often overlooking color and contextual information. Exploiting these nonspatial attributes for 3D geospatial object detection thus becomes crucial. Our study pioneers explicit incorporation of spatial autocorrelation of color information into 3D deep learning for object detection. We introduce an innovative framework to estimate spatial autocorrelation, addressing challenges in unstructured 3D data sets. Our experiments suggest the effectiveness of incorporating spatial autocorrelation features in enhancing the accuracy of 3D deep learning models for geospatial object detection. We further investigate the uncertainty of such contextual information brought by diverse configurations, exemplified by the number of nearest neighbors. This study advances 3D geospatial object detection via using spatial autocorrelation to inform deep learning algorithms, strengthening the connection between GIScience and artificial intelligence and, thus, holding implications for diverse GeoAI applications.