CAGIS Seminar 10/20/2022, 2:30: Exploring Human Mobility using Wi-Fi Log Data Processing and Spatial-temporal Analysis | Zhu
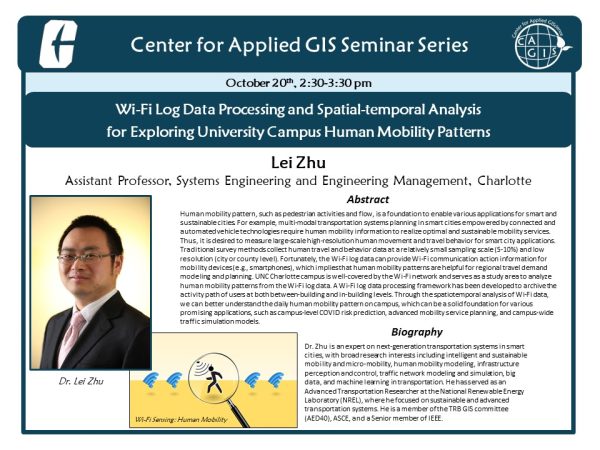
Update: A recording of this presentation is avaliable at the CAGIS YouTube Channel: https://www.youtube.com/playlist?list=PLfoz4aBkHaMxbhGhO_UZ_Nu9Mujnk_bsU Our upcoming CAGIS Seminar features a practical application of existing technologies to generate novel ways of mapping human activity. On Thursday, October 20th at 2:30*, Charlotte Assistant Professor Lei Zhu will present:
Wi-Fi Log Data Processing and Spatial-temporal Analysis for Exploring University Campus Human Mobility Patterns
Abstract
Human mobility patterns, such as pedestrian activities and flow, is a foundation to enable various applications for smart and sustainable cities. For example, multi-modal transportation systems planning in smart cities empowered by connected and automated vehicle technologies require human mobility information to realize optimal and sustainable mobility services. Thus, it is desired to measure large-scale high-resolution human movement and travel behavior for smart city applications. Traditional survey methods collect human travel and behavior data at a relatively small sampling scale (5-10%) and low resolution (city or county level). Fortunately, the Wi-Fi log data can provide Wi-Fi communication action information for mobility devices (e.g., smartphones), which implies that human mobility patterns are helpful for regional travel demand modeling and planning. UNC Charlotte campus is well-covered by the Wi-Fi network and serves as a study area to analyze human mobility patterns from the Wi-Fi log data. A Wi-Fi log data processing framework has been developed to archive the activity path of users at both between-building and in-building levels. Through the spatiotemporal analysis of Wi-Fi data, we can better understand the daily human mobility pattern on campus, which can be a solid foundation for various promising applications, such as campus-level COVID risk prediction, advanced mobility service planning, and campus-wide traffic simulation models.
Dr. Zhu is an expert on next-generation transportation systems in smart cities, with broad research interests including intelligent and sustainable mobility and micro-mobility, human mobility modeling, infrastructure perception and control, traffic network modeling and simulation, big data, and machine learning in transportation. He has served as an Advanced Transportation Researcher at the National Renewable Energy Laboratory (NREL), where he focused on sustainable and advanced transportation systems. He is a member of the TRB GIS committee (AED40), ASCE, and a Senior member of IEEE.